Last week, the article “Pentagon’s AI chief says data labeling is key to win race with China” caught my eye. The title tells you almost everything you need to know. The Pentagon believes that success in AI depends on collecting and labeling mountains of data.
If only it were that simple. AI implementations the military will be developing are not well established and understood technical challenges like recommender systems or chat bots. The military will be developing AI for unconventional sensors where pre-trained models are of limited use and clean labeled data is a persistent obstacle.
There is no practical way to label a multi-sensor dataset at scale. Here is a simple example. Assume you are labeling sensor data that measures ambient temperature and humidity for a room. How easy is it to label the point in the temperature graph where the HVAC turned on or off? Now add a second sensor to the mix. For both temperature and humidity combined, where did the HVAC turn on or off?
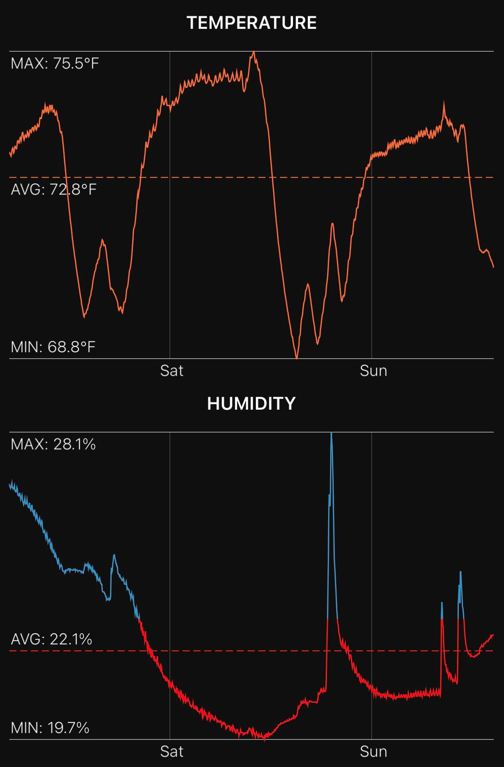
The absence of labeled data in multi-physics sensor domains steers AI from conventional supervised learning toward self-supervised (e.g., autoregressive) learning and semi-supervised learning.The AI team needs to be prepared to rely less on supervised learning because they will not have enough quality labeled data – at every step. Finally, it is important to experiment with unsupervised learning approaches, but keep in mind that this technique alters what stakeholders should expect from AI.